Skills: Prompt Engineering, API Calls, Data Science, Sequence2Sequence Models
Tools/Packages used: Python, Mistral 7B, Chroma DB, Unstructured Bricks
Link for code: Github
What you will do:
Build a LLM powered Retrieval Augmented Generation pipeline that allows you to quickly obtain answers from information that ChatGPT and other pre-trained LLM's don't know about
Introduction:
LLM's have solved many problems but one of the biggest drawback with any state of the art LLM is its knowledge cap based on the data it was trained on. This isn't to say that pre-trained LLM's aren't clever, they surely are creative enough to 'invent' un-true information which can pass of as extremely well written fiction but hazardous when used in situations where the exact information is required.
Like in the case of medicine, where according to a study by the NCBI, when prompting ChatGPT to provide medical papers with references it was found that 47% of the medical references generated were made up references and while 46% were real they had inaccuracies in their details. Only 7% of the references were completely accurate and true. The process for retraining a fine-tuned model is expensive and doesn't really improve its performance in other metrics like quality of output. It's only the factual accuracy which really is affected when you have a model trained on outdated data, but this can be easily fixed by providing it 'context' which asks the model to correct itself with the right results
Sort of like a cheat sheet in an open book exam. The best part about this is, you can make your own solution for this using free open source tools, so the next time you have a problem that needs accurate and up to date information to be returned by your model try this out!
Tools required:
-
Python: Basic Python programming, API calling and Data Science knowledge,
-
LLM: Mistral 7B
-
ChromaDB: Vector-store Database package, easily installable in python using pip (doesn't support Python 3.10) 🔗
-
Unstructured Bricks: Document parsing package with many many options! 🔗
My Solution:
Using a highly modular and flexible pipeline that is simple to implement, replicate and scale if needed. I will not use LangChain here as my original goal was to use to not use LangChain at all and directly implement the vanilla tools/packages for the solution.
Overview of the Solution
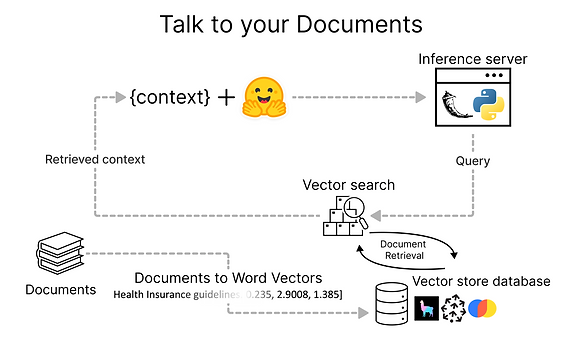.png)